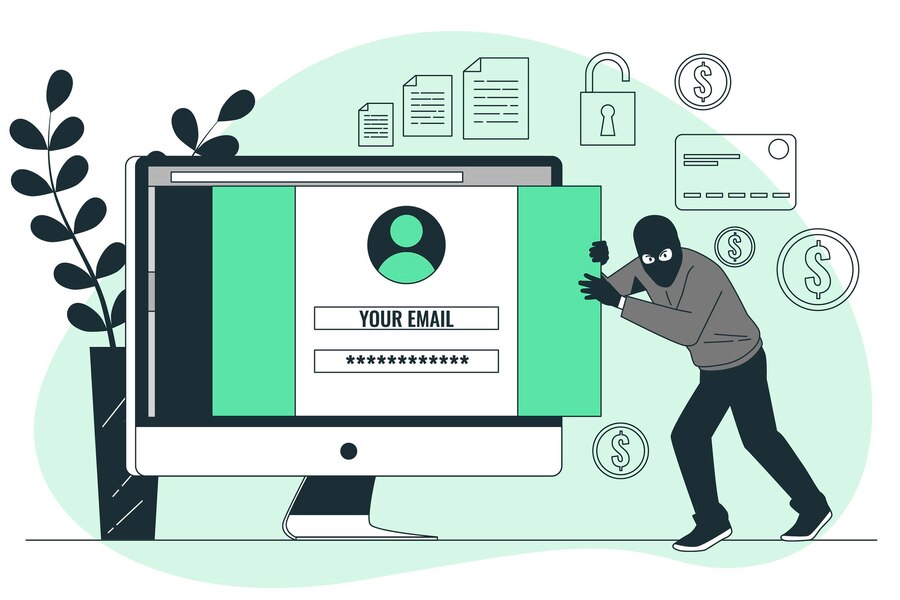
AI in Fraud Detection: Enhancing Security in Financial Transactions
In today’s digital age, financial transactions are increasingly susceptible to fraud due to the rise of online banking and e-commerce. As cybercriminals become more sophisticated, financial institutions are turning to artificial intelligence (AI) and machine learning (ML) to enhance their fraud detection capabilities. This blog post explores how these technologies are being utilized to identify suspicious activities and prevent fraud in real-time.
The Role of AI and Machine Learning in Fraud Detection
AI and ML play a crucial role in modern fraud detection systems. These technologies enable financial institutions to analyze vast amounts of transaction data quickly and accurately, identifying patterns that may indicate fraudulent behavior. The main techniques employed include:
- Anomaly Detection: This method involves establishing a baseline of normal behavior for users and transactions. Any deviations from this norm—such as unusual transaction amounts or locations—are flagged for further investigation.
- Behavioral Analysis: By analyzing historical transaction data, AI models can predict typical behavior patterns for customers, merchants, devices, and accounts. This allows for the identification of suspicious activities that deviate from established norms.
- Predictive Analytics: AI systems use historical data to train models that can predict future fraudulent activities. This proactive approach allows institutions to act before fraud occurs.
How AI Enhances Fraud Detection
The integration of AI into fraud detection processes offers several significant benefits:
- Real-Time Processing: AI algorithms can process incoming data in milliseconds, allowing for immediate action against potential threats. This speed is critical in preventing fraudulent transactions before they are completed.
- Reduced False Positives: Traditional fraud detection methods often generate numerous false positives, leading to customer dissatisfaction and unnecessary investigations. AI systems continuously learn from new data, improving their accuracy and reducing the number of false alarms.
- Scalability: As transaction volumes grow, the need for efficient analysis increases. AI systems can handle vast datasets without a decrease in performance, making them ideal for financial institutions experiencing rapid growth.
- Cost Efficiency: Automating fraud detection reduces the need for extensive manual reviews, saving time and resources while enhancing overall operational efficiency.
Machine Learning Models in Action
Machine learning models are at the forefront of fraud detection technology. They utilize various algorithms to identify suspicious patterns and behaviors:
- Supervised Learning: This approach uses labeled datasets (historical transactions marked as legitimate or fraudulent) to train models that can classify new transactions accordingly.
- Unsupervised Learning: In contrast, unsupervised learning analyzes data without pre-existing labels, allowing the model to identify hidden patterns or anomalies that may indicate fraud.
- Deep Learning: Advanced deep learning techniques enable the analysis of complex datasets with multiple layers of abstraction, improving the system’s ability to detect sophisticated fraud schemes.
Real-World Applications
Numerous financial institutions have successfully implemented AI-driven fraud detection systems:
- Citibank has leveraged natural language processing (NLP) to significantly reduce phishing attacks by 70%, showcasing how AI can enhance security measures beyond traditional methods.
- Walmart has utilized real-time video analysis powered by AI to decrease shoplifting incidents by 25%, demonstrating the versatility of these technologies across different sectors.
- In Vietnam, FE Credit, a subsidiary of VPBank, saved over $15 million through proactive fraud prevention measures enabled by HyperVerge’s AI solutions.
Challenges and Future Directions
While AI presents substantial advantages in combating financial fraud, challenges remain:
- Data Privacy Concerns: The use of personal data for training models raises privacy issues that must be addressed through robust data protection policies.
- Evolving Threats: Cybercriminals continuously adapt their tactics, necessitating ongoing updates and improvements to AI models to keep pace with new forms of fraud.
- Integration with Legacy Systems: Many financial institutions still rely on outdated systems that may not easily integrate with advanced AI technologies. Transitioning to modern infrastructures is essential for maximizing the benefits of AI in fraud detection.
Conclusion
AI and machine learning are transforming the landscape of financial fraud detection by providing enhanced accuracy, speed, and efficiency. As financial institutions increasingly adopt these technologies, they not only improve their ability to combat fraud but also enhance customer trust and satisfaction. The ongoing evolution of these technologies will be crucial in staying ahead of sophisticated cyber threats in an ever-changing digital environment.
For further reading on this topic, you can explore the following resources: