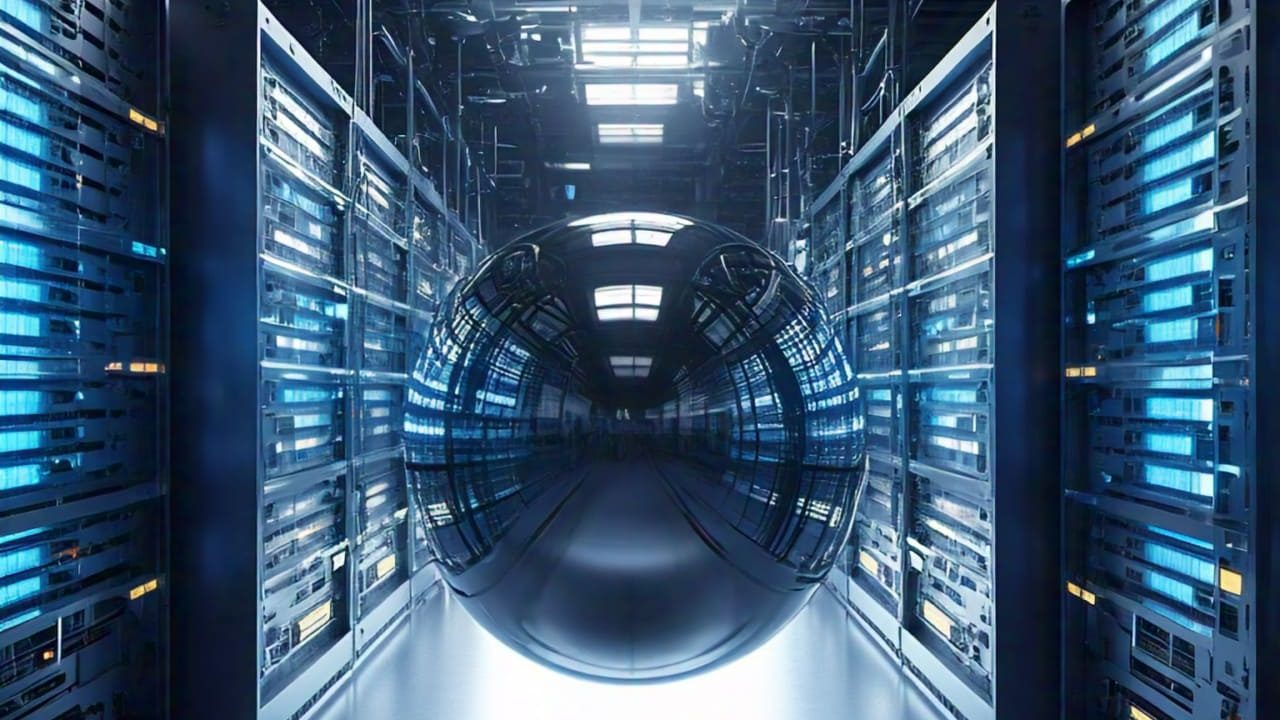
AI’s Data Drain: What’s Next?
The internet has undergone a significant transformation in recent years, with the rise of artificial intelligence (AI) and machine learning (ML) technologies. The increasing demand for data to train and power these AI systems has led to a phenomenon known as the “Great Data Famine.” In this blog, we will explore the causes and consequences of the Great Data Famine, and what it means for the future of the internet.
What is the Great Data Famine?
The Great Data Famine refers to the scarcity of high-quality data that is needed to train and power AI systems. As AI technologies continue to advance, the demand for data has increased exponentially, leading to a shortage of available data. This shortage has significant implications for the development and deployment of AI systems, as well as the future of the internet.
Causes of the Great Data Famine
There are several causes of the Great Data Famine, including:
- Increasing Demand for Data: The increasing demand for data to train and power AI systems has led to a shortage of available data. As AI technologies continue to advance, the demand for data is expected to increase even further.
- Lack of Data Standardization: The lack of standardization in data collection and storage has made it difficult to share and reuse data, leading to a shortage of available data.
- Data Quality Issues: The quality of available data is often poor, with many datasets containing errors, biases, and inconsistencies. This can make it difficult to train and power AI systems effectively.
Consequences of the Great Data Famine
The Great Data Famine has significant consequences for the development and deployment of AI systems, as well as the future of the internet. Some of the consequences include:
- Reduced Accuracy of AI Systems: The shortage of high-quality data can reduce the accuracy of AI systems, making them less effective and reliable.
- Increased Costs: The shortage of data can increase the costs of developing and deploying AI systems, making them less accessible to smaller organizations and individuals.
- Delayed Adoption of AI Technologies: The Great Data Famine can delay the adoption of AI technologies, as organizations may be hesitant to invest in AI systems that are not effective or reliable.
Real-World Examples of the Great Data Famine
The Great Data Famine is not just a theoretical concept, but a real-world problem that is affecting many organizations and industries. Some examples include:
- Self-Driving Cars: The development of self-driving cars requires large amounts of high-quality data, including images, videos, and sensor readings. However, the shortage of data has made it difficult to train and deploy self-driving cars effectively.
- Medical Diagnosis: The development of AI systems for medical diagnosis requires large amounts of high-quality data, including medical images and patient records. However, the shortage of data has made it difficult to train and deploy these systems effectively.
- Customer Service Chatbots: The development of customer service chatbots requires large amounts of high-quality data, including customer interactions and feedback. However, the shortage of data has made it difficult to train and deploy these chatbots effectively.
Solutions to the Great Data Famine
There are several solutions to the Great Data Famine, including:
- Data Sharing and Collaboration: Organizations can share and collaborate on data to increase the availability of high-quality data.
- Data Standardization: Standardizing data collection and storage can make it easier to share and reuse data, reducing the shortage of available data.
- Synthetic Data Generation: Generating synthetic data can help to supplement the shortage of real-world data, making it easier to train and deploy AI systems.
Conclusion
The Great Data Famine is a significant challenge that is affecting the development and deployment of AI systems. However, by understanding the causes and consequences of the Great Data Famine, we can work towards solutions that increase the availability of high-quality data. As AI technologies continue to advance, it is essential that we prioritize data sharing, standardization, and generation to ensure that AI systems are effective, reliable, and accessible to all.
References
- The Great Data Famine: How AI Ate the Internet and What’s Next by Sify.com
- The Challenges of AI-Driven Decision Making by Harvard Business Review
- The Importance of Data Quality in AI Development by Data Science Central