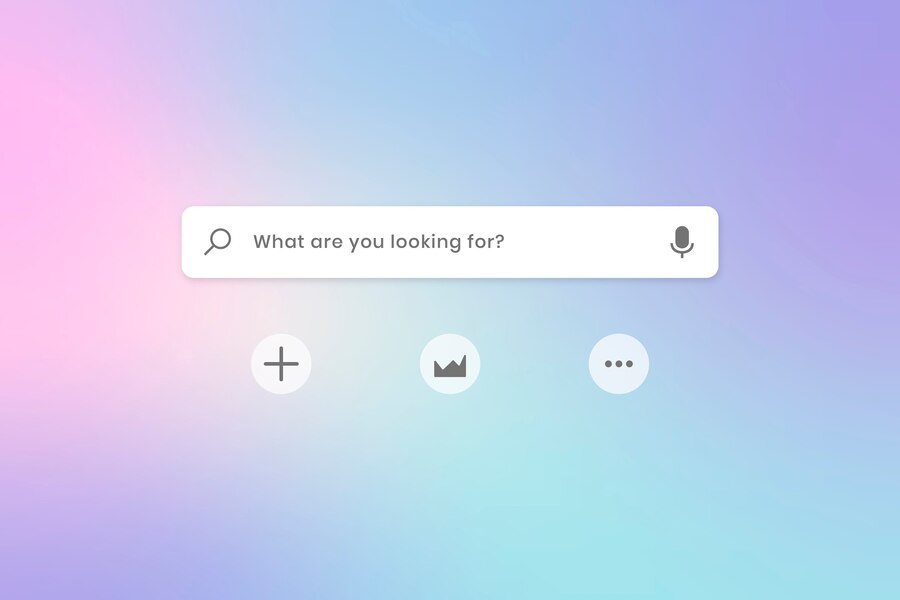
The Accuracy of AI Web Searches: Separating Fact from Fiction
The rise of artificial intelligence (AI) has transformed the way we interact with the internet. One of the most significant advancements in AI is the development of AI-powered web searches, which promise to revolutionize the way we find information online. But how accurate are these AI web searches? In this blog, we will delve into the world of AI web searches, exploring their capabilities, limitations, and potential applications.
What are AI Web Searches?
AI web searches use machine learning algorithms to analyze and understand the context of a search query, providing more accurate and relevant results. Unlike traditional search engines, which rely on keyword matching and ranking algorithms, AI web searches use natural language processing (NLP) and machine learning to understand the intent behind a search query.
How Do AI Web Searches Work?
AI web searches work by combining several technologies, including:
- Natural Language Processing (NLP): NLP is used to analyze and understand the context of a search query, identifying the intent and meaning behind the words.
- Machine Learning: Machine learning algorithms are used to analyze the search query and provide relevant results based on the user’s intent.
- Knowledge Graphs: Knowledge graphs are used to store and retrieve information about entities, relationships, and concepts, providing a deeper understanding of the search query.
The Accuracy of AI Web Searches
The accuracy of AI web searches depends on several factors, including:
- Quality of Training Data: The quality of the training data used to train the AI algorithm has a significant impact on the accuracy of the search results.
- Complexity of the Search Query: The complexity of the search query can affect the accuracy of the search results, with more complex queries requiring more advanced algorithms.
- Contextual Understanding: The ability of the AI algorithm to understand the context of the search query is critical to providing accurate results.
Use Cases for AI Web Searches
AI web searches have a wide range of applications, including:
- Enterprise Search: AI web searches can be used to improve the accuracy and relevance of search results within an enterprise, providing employees with faster access to information.
- E-commerce Search: AI web searches can be used to improve the accuracy and relevance of search results on e-commerce websites, providing customers with faster access to products.
- Healthcare Search: AI web searches can be used to improve the accuracy and relevance of search results in healthcare, providing medical professionals with faster access to information.
Real-World Examples of AI Web Searches
Here are some real-world examples of AI web searches in action:
- Google’s Knowledge Graph: Google’s Knowledge Graph is a knowledge graph that stores information about entities, relationships, and concepts, providing users with more accurate and relevant search results.
- Bing’s Entity Search: Bing’s Entity Search is a search engine that uses AI to understand the context of a search query, providing users with more accurate and relevant results.
- Amazon’s Product Search: Amazon’s Product Search is a search engine that uses AI to understand the context of a search query, providing customers with more accurate and relevant product recommendations.
Benefits of AI Web Searches
AI web searches offer several benefits, including:
- Improved Accuracy: AI web searches can provide more accurate and relevant results, reducing the time and effort required to find information.
- Increased Efficiency: AI web searches can automate the search process, freeing up human resources for more strategic tasks.
- Enhanced User Experience: AI web searches can provide a more personalized and intuitive search experience, improving user satisfaction and engagement.
Challenges and Limitations of AI Web Searches
While AI web searches offer several benefits, they also have several challenges and limitations, including:
- Bias and Error: AI web searches can be biased and error-prone, providing inaccurate or irrelevant results.
- Lack of Transparency: AI web searches can lack transparency, making it difficult to understand how the search results were generated.
- Dependence on Data Quality: AI web searches depend on the quality of the training data, which can be limited or biased.
Conclusion
AI web searches have the potential to revolutionize the way we find information online. By combining NLP, machine learning, and knowledge graphs, AI web searches can provide more accurate and relevant results, improving the user experience and increasing efficiency. However, AI web searches also have several challenges and limitations, including bias and error, lack of transparency, and dependence on data quality. As AI web searches continue to evolve, it is essential to address these challenges and limitations to ensure that they provide accurate and relevant results.